New developments in analyzing data
A perspective article by Dr. Jingyi Jessica Li, associate professor of statistics, was published in the journal Patterns, a new data science journal of Cell Press. The article can be read here.
Co-authored by Dr. Xin Tong at University of Southern California, Dr. Li’s article offers practical guidelines for choosing between two widely-used data analytical strategies – hypothesis testing and binary classification – for making binary decisions from data.
Although both strategies are major topics taught in undergraduate statistics classes, they are rarely contrasted with one and another, and thus their distinctions are often ambiguous to data science students, practitioners, and even researchers. In particular, it can be perplexing whether a real-world problem should be formulated as a hypothesis testing problem or a binary classification task.
A prominent example is the computational prediction of cancer driver genes, for which computational tools were developed using both formulations; however, the two formulations are not both appropriate.
To address this issue, Dr. Li’s article explains conceptual differences between hypothesis testing and binary classification while also providing practical guidelines in a concrete data analysis setting. Further, her article explains why binary classification is more appropriate than hypothesis testing is for cancer driver gene prediction. Given the wide applications of hypothesis testing and binary classification, Dr. Li’s article is expected to be of broad interest to students and researchers in statistics, data science, and related disciplines.
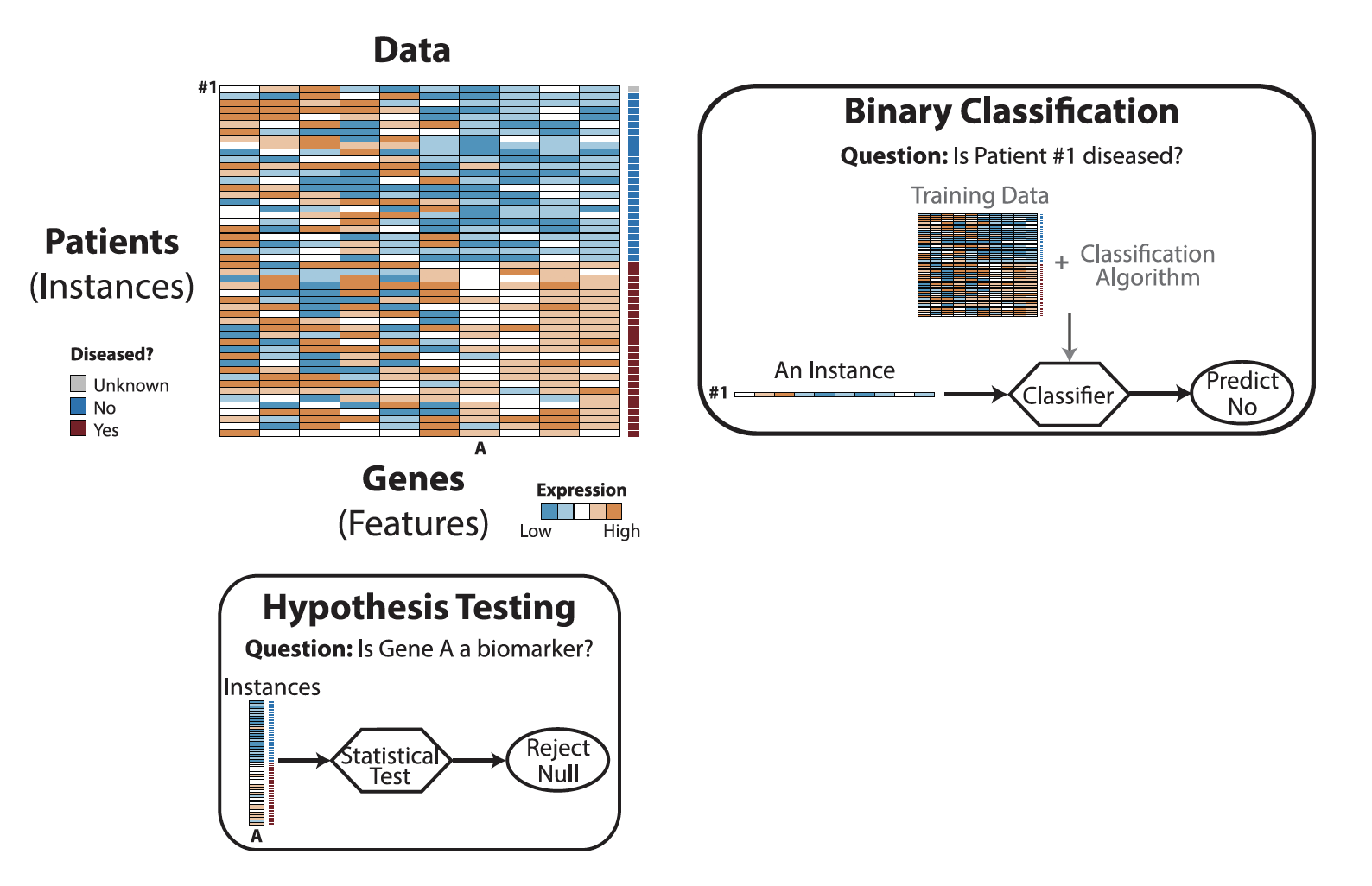
Hypothesis testing uses all the available instances to address a feature-related question: is a gene a biomarker with different expression levels in healthy and diseased patients? Binary classification answers an instance-related question: is a patient diseased?
In conjunction with this perspective article, Dr. Li and her Ph.D. students Yiling Chen and Xinzhou Ge collaborated with Dr. Wei Li’s lab at University of California, Irvine, in developing a computational algorithm called DORGE to predict cancer driver genes from the most comprehensive collection of genomics and epigenomics data.
The team’s article about DORGE was published in Science Advances, a multi-disciplinary journal of the American Association for the Advancement of Science. In the DORGE algorithm, Dr. Li and co-authors formulated the cancer driver gene prediction task as a binary classification problem, and their results show that DORGE outperformed existing prediction algorithms in multiple aspects.
Overall, their study has deepened the understanding of epigenetic mechanisms in tumorigenesis and revealed a previously undetected repertoire of cancer driver genes.
Read more about Dr. Li’s research on her group website.
Tags: News